TDAI supports core faculty (TDAI hires) with Core Faculty Catalyst Grants of up to $10,000 to initiate, advance, and disseminate interdisciplinary research that has the potential to benefit the greater good. Congratulations to all Catalyst Grant recipients.
If you are wondering how to apply to receive this grant, you can find out more information here.
2024-25 Catalyst Project Grants
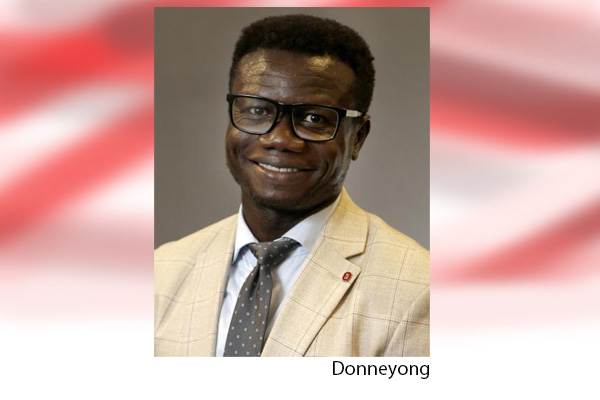
Dr. Macarius Donneyong (Pharmacy Practice and Science)
As the intersection of artificial intelligence (AI) and epidemiology gains prominence, there is a pressing need for a collaborative dialogue between academics and state public health workers to leverage AI's transformative potential to improve public health. However, there is a disconnect between AI research and practical application by public health professionals. Thus, we have planned a special session during the 2024 American College of Epidemiology (ACE) national conference to bring together academics and public health professionals to share experiences and to collaborate on how to better develop and use AI for public health good. This special session dubbed the All-Ohio session, aligns with the theme of the 2024 ACE annual conference, “Integrating Epidemiology and Artificial Intelligence to Advance Public Health.” The targeted attendees of the All-Ohio session will be local faculty, staff, and students from academic institutions within the Ohio region and public health professionals. This session will feature a plenary talk, break-out oral presentations, poster presentations, and a networking session. The scientific talks will focus on current AI methodologies, case studies, and best practices while addressing regulatory, ethical, and data privacy concerns.
The potential benefits of the All-Ohio session are innumerable. For instance, by bringing together academics who are pioneering AI research and public health practitioners, who are the boots on the ground for improving the health of all, the All-Ohio session could potentially lay the foundation for the application of AI for public health good. Furthermore, we believe that participants will develop collaborative partnerships within and across both academics and public health professionals to foster AI research and implementation. By funding the All-Ohio session, TDAI would be playing a critical role as the catalyst for a new academia-public health alliance in the advancement of AI research and application for public health goods, especially in the local Ohio region.
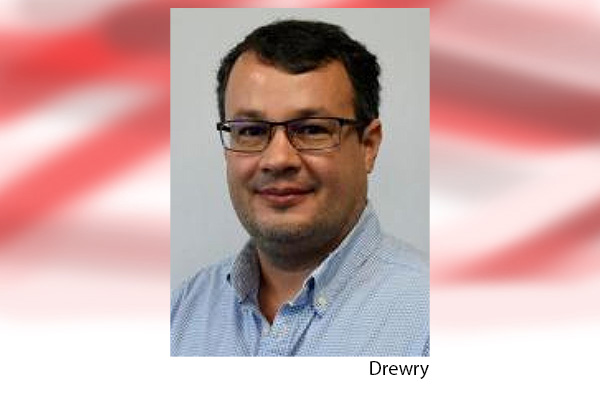
Darren Drewry (Agricultural Engineering)
Legumes are the major source of protein in the developing world where animal-based protein sources are limited and are central to the emerging plant-based food industry. Phaseolus beans play an important nutritional and economic role in the United States and throughout the world, largely driven by their high protein content, amino acid profiles that are complementary to cereals, and by providing a significant source of fiber, vitamins, and other micronutrients. This project will facilitate a proof-of-concept demonstration of the application of high-throughput phenotyping methods to quantify the nutritional resources held in gene banks. We will leverage an existing spectral library consisting of hyper-spectral reflectance (HSR) measurements made across 1600 bean accessions maintained at the USDA common bean gene bank in Pullman, Washington. This funding will be used to acquire wet chemistry assays of total protein and fiber contents for a subset of accessions of Phaseolus vulgaris and Phaseolus lunatus, common and lima bean, respectively, providing a basis to test existing algorithms and develop new algorithms to relate HSR to seed protein and fiber content. We will likewise use this funding to genotype these accessions, providing an understanding of the genetic architecture associated with seed protein and fiber contents and relating HSR-based traits to underlying genetic diversity. This work will allow us to open the door to understanding the nutritional diversity contained in major global gene banks, advancing selection and breeding efforts to enhance food and nutrition security.
2023-24 Catalyst Project Grants
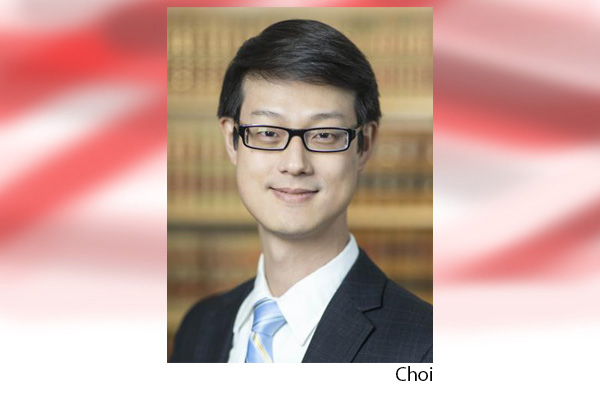
Bryan Choi (Computer Science and Engineering)
The prevalence of sophisticated computation and massive data collection has created a growing need for research that combines a deep understanding of the power and the limitations of computing with expertise in multiple aspects of law. Indeed, recent years have seen multiple venues for disseminating such research. These include, among others, the Symposium on the Foundations of Responsible Computing, the ACM Conference on Artificial Intelligence, Ethics, and Society, the ACM Conference on Fairness, Accountability, and Transparency, the 2020 Simons Institute Workshop on Algorithm Design, Law, and Policy, and the 2020 DIMACS workshop on Co-Development of Computer Science and Law. The Computer Science and Law Symposium (whose first and second meetings took place in 2019 and 2022, respectively) seeks to build a community of scholars, as well as lawyers and computing professionals, who are fluent both in computational thinking and its rigorous mathematical formalisms, and in legal scholarship and thought with its equally rigorous yet human-centric set of principles, methodologies, and goals. Central to the study of “computer science and law” is the creation of a body of scholarship aimed towards the co-design of law and computing technology to further society.
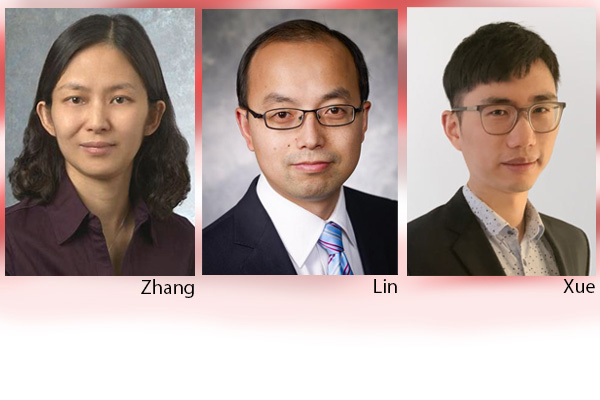
Xinmiao Zhang (Electrical & Computer Engineering), Zhiqiang Lin (Computer Science & Engineering), and Yuan Xue (Medicine)
Ensuring the security and privacy of electronic health records (EHRs) in the digital age is paramount. Traditional de-identification methods, though widely implemented, often lead to loss of information, degrading the performance of predictive models. This project proposes to utilize the new homomorphic encryption (HE) technique, which allows computations to be directly carried out on encrypted data, to achieve secure and private analytics on healthcare data.
Our goal is to significantly boost the speed of HE by orders of magnitude by taking into account the specifics of computations involved in neural networks (NNs) and data analytics. By integrating algorithmic reformulations with hardware architecture design, we aim to achieve practical privacy-preserving data analytics and NNs. This research has profound implications for applications that need to preserve user data privacy over the cloud, such as data analytics over sensitive information, medical diagnosis using patient images, genome sequencing, and beyond.
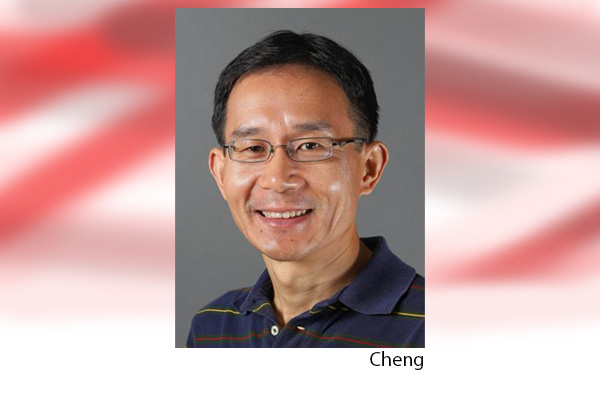
Xiaolin Cheng (Pharmacy)
Peptides are essential in discovering antibiotics and designing biomaterials, but creating peptides that precisely inhibit specific protein-protein interactions (PPIs) is a big challenge. In this study, we introduce a new way to design peptides targeting specific proteins. Our method uses the binding mode of known peptides as a template, combining a Gated Recurrent Unit (GRU)-based variational autoencoder (VAE) with Rosetta FlexPepDock for creating peptide sequences and assessing how well they bind. Molecular dynamics (MD) simulations then refine the selection of peptides for experimental testing. We applied this method to design peptide inhibitors for β-catenin, resulting in promising outcomes. Among the 12 designed peptides, six (50%) showed improved binding compared to their parent peptides. Notably, the best-designed peptide, CAL-2, demonstrated a 15-fold improvement in binding to β-catenin. To demonstrate the broad applicability of our peptide generative models, we plan to extend its use to a more challenging system: the NF-κB essential modulator (NEMO). NF-κB is a key player in activating genes related to inflammation, immune response, and cell survival. These results will demonstrate how our peptide generative models can be applied to different protein systems, highlighting the successful integration of deep learning and structure-based modeling and simulation for designing targeted peptides. Our research endeavors to advance the field of deep learning-based molecular design, offering innovative solutions to target specific protein-protein interactions with implications for therapeutic development.
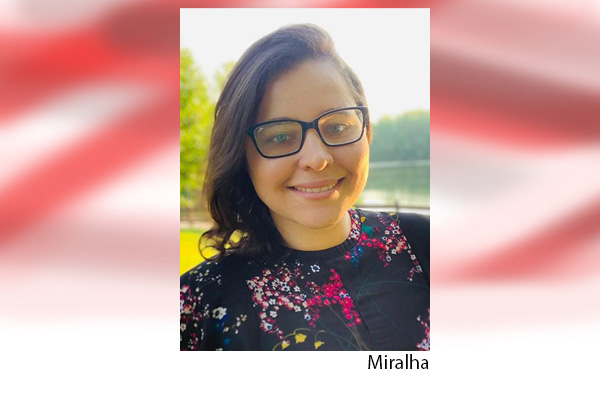
Lorrayne Miralha (Sustainability Institute)
As specialty crops, floriculture and nurseries have become a fast-growing industry, with US sales growing 16% from 2019-2022 - totaling $6.7 billion in 2022 (NASS, 2023). Representing the North Central region (NCR), Ohio, Michigan, and Wisconsin are among the top 10 largest states dominating the floriculture and nursery market. With the unpredictable climate scenario, the demand for irrigation of these crops has risen significantly, posing threats to growers and the environment. Prior research has already identified that growers are interested in improving their water resources management strategies due to expected production, environmental, and economic challenges (White et al., 2019). Specifically, stakeholders were interested in understanding how water quality relates to the plant health conditions observed in their operations and how they can use their nutrient-rich water to their economic advantage. Given the related issues, developing sustainable strategies to adapt to changing climate conditions, optimize water usage, minimize the industry’s ecological footprint, and maximize growers’ profits is critical. More critically, no study has yet quantified this industry's hydrological and economic signatures across scales in the NCR. Our project will map climate-vulnerable floriculture and nursery industry locations, quantify their hydrological and economic trends, and develop long-term relationships with stakeholders for the economic viability and environmental sustainability of specialty crop systems. An interdisciplinary team with skills in hydrology, climate, extension, specialty crops, and economics is needed to achieve these goals. The outcomes of this proposal will bring insights into strategies for targeted management considering climate uncertainty and water sustainability in specialty crop systems.
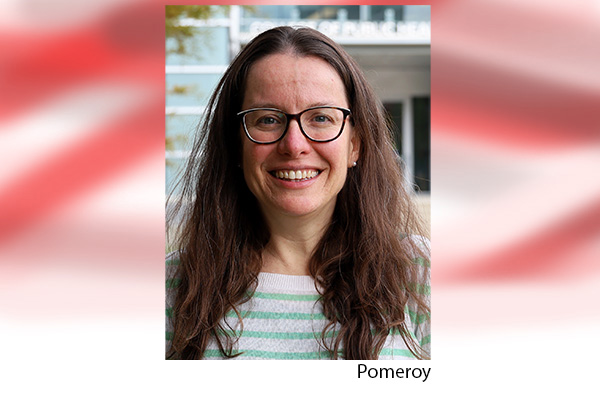
Laura Pomeroy (Public Health)
A pandemic of avian influenza virus (AIV) subtype H5N1 has been occurring since 2020 in Europe, Asia, and Africa. The virus then spread to North America in 2021 and South America in 2022. To date, over 9,000 wild birds with infections have been detected in the US and over 90 million poultry have been affected. Recently, 36 cattle herds have confirmed infection across 9 states. Much is known about influenza viral genetics and host immunity but less is known about disease and animal ecology of host species. A better understanding of AIV disease ecology and identification of avian communities that participate in AIV transmission can inform control decisions and have a positive societal impact. Models of infectious disease dynamics can be useful, but we lack knowledge about a key parameter that quantifies contact among birds. To inform, we propose to collect data using two different devices – one that records images and one that records audio – and compare them to observations of an expert bird identifier (“birder”) to quantify the rate of contact among different bird species. This will allow us to quantify (1) counts of birds observed, by species, obtained by different methods at different times, (2) contact rates among species, and (3) how our observation methods might bias our counts. The next steps include an NSF grant proposal submission using observations collected as preliminary data, submitting two manuscripts resulting from this research, and using the equipment purchased with this grant in other locations to gather additional data. Finally, we will use obtained contact rate data in models of AIV dynamics.
2022-23 Catalyst Project Grants
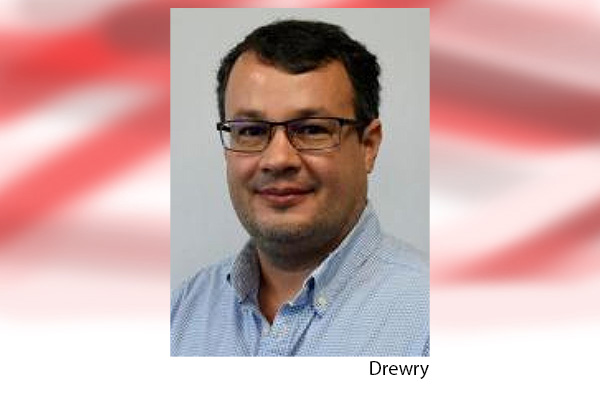
Darren Drewry (Agricultural Engineering)
Legumes are the major source of protein in the developing world where animal-based protein sources are limited and are central to the emerging plant-based food industry. Phaseolus (common) beans play an important nutritional and economical role in the United States and throughout the world, largely driven by their high protein content, amino acid profiles that are complementary to cereals, and by providing a significant source of fiber, vitamins, and other micronutrients. This project will allow for an initial proof-of-concept study of the application of hyper-spectral reflectance measurements to model common bean nutritional traits. This work will be conducted at the International Center for Tropical Agriculture (CIAT) near Cali, Columbia, where the world’s largest common bean seed bank is maintained. This initial effort will allow us to expand on our work to model common bean protein content and seed size with hyper-spectral reflectance applied on a broader set of nutritional traits analyzed at laboratories at CIAT. This work will open the door to understanding the nutritional diversity contained in the CIAT seed bank and associated seed banks in the US and other countries, advancing selection and breeding efforts to enhance food and nutrition security.
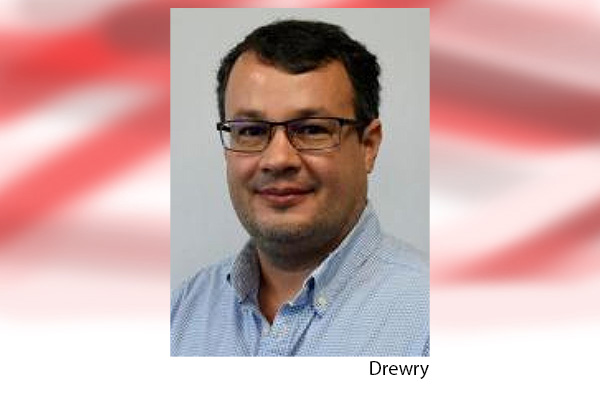
Darren Drewry (Agricultural Engineering)
Understanding vegetation water use has broad societal utility in areas such as predicting agricultural production in current and future climate regimes, estimating resource needs in emerging areas of agricultural production such as controlled environment agriculture, and the development of more robust plant varieties. Despite the central importance of estimating vegetation water use and stress, observations of plant physiological parameters related to this process are constrained by being very low throughput (i.e. several minutes per measurement) and non-continuous in time (requiring a human to conduct the observations) and often require destructive measurement. A new approach to estimating leaf stomatal response to environmental variations has been developed and validated and is available to the research community. This request to purchase one of these “plant wearable sensor” systems will allow us to open new doors to model development and validation in domains spanning field and controlled environment agricultural production.
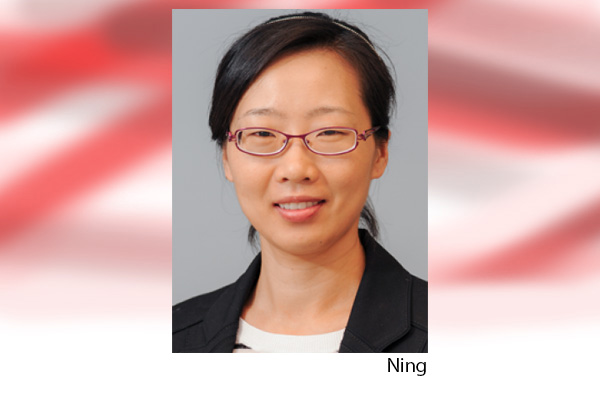
Xia Ning (Computer Science & Engineering)
We are organizing a two-day, 2023 Advanced Computational Neuroscience Network (ACNN) Workshop on Big Neuroscience Data, Tools, Protocols & Services to take place in September 2023 at The Ohio State University. We are applying for this Pilot Award to support speaker travel costs, support fees, and other conference logistics. This two-day conference is focused on working with high-density human data—spatial information, temporal data from physiological sensors or phones, for example—and integrating that with the large, publicly available neuroimaging datasets collected on a very different time scale. The targeted audience is graduate students and trainees in various fields to give them experience with the tools and approaches to work with deeply phenotyped data. The primary question we are addressing is the need to facilitate the integration of data across multiple scales and biomedical disciplines to build the best predictive models from the wealth of data while simultaneously being precise in personalized medicine.
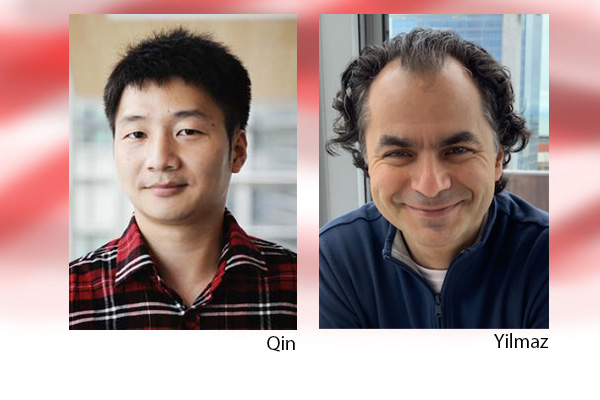
Rongjun Qin (Civil, Environmental, & Geodetic Engineering), Alper Yilmaz (Civil, Environmental, & Geodetic Engineering)
We propose to support the ISPRS (International Society for Photogrammetry and Remote Sensing), technical commission II, for its online webinar series that will reach roughly more than 3,000 participants, including renowned experts and scientists, faculties, graduate students worldwide focusing on imaging sciences. The webinar will be held roughly every other month, inviting speakers for technical talks and career talks (targeted at graduate students and young scientists who will become future leaders of the field). The webinar will significantly increase the OSU’s and TDAI’s visibility over such an event in leading and supporting international commissions/organizations that will influence the research field and the next generations worldwide.
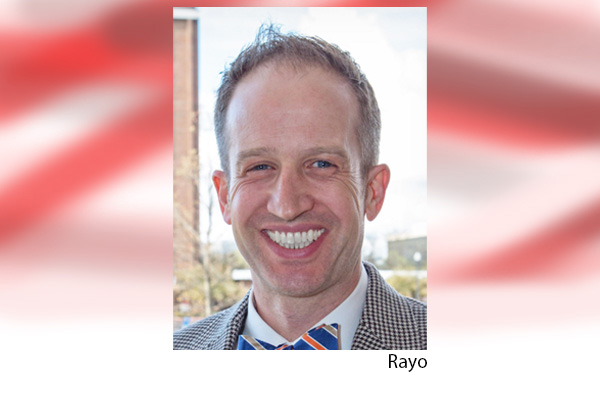
Mike Rayo (Integrated Systems Engineer)
We are proposing an unobtrusive data collection method, Joint Activity Monitoring (JAM), that will facilitate both current and proactive assessments of systems performance. Being proactive is preferred over reactiveness in many high-consequence domains1,2, as solving past problems does not guarantee to be able to address future surprises. Proactivity, which enables anticipation and successful adaptation 3, is an essential ingredient of systems resilience4. In order to provide the readiness to respond, especially prior to disturbances, a resilient system needs to continuously monitor its performance and the environment to look for threats in the near future5. However, there is not an abundance of practical applications that equip systems with this monitoring capability that provides anticipatory insights into how systems can sustain performance in the face of potential challenges6,7. We are developing Joint Activity Monitoring (JAM) to address the current gap in the industry: we are currently working with the department of epidemiology in a tertiary care hospital to instrument a JAM-enabled decision-support tool designed to facilitate the detection, confirmation, and anticipation of hospital-acquired infections (HAI) clusters. JAM is an extension of our Joint Activity Testing (JAT) simulation method8 but applied to work systems in situ. JAM will provide an unobtrusive monitoring platform that continuously collects operational data around system performance relative to current challenges. Anticipatory insights on future behaviors can be generated by analyzing how the system responds to day-to-day demands, and proactive behaviors can be initiated prior to the occurrence of disturbing events that may otherwise lead to significant system disruptions that impact patient safety.
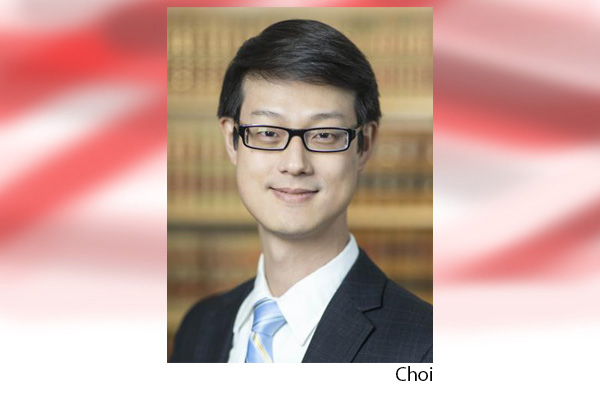
Bryan Choi (Computer Science & Engineering)
The rapid development of AI technologies and their increasing ubiquity in society are transforming many aspects of our day-to-day lives and revolutionizing a range of domains such as transportation and healthcare. The widespread use of AI systems in society, however, has given rise to serious concerns about accountability, fairness, privacy, safety, and transparency. In recent years, there has been a growing body of AI research devoted to tackling these issues. Meanwhile, the regulation of AI technologies has been drawing increasing attention from legal experts and policymakers. For example, the European Commission published a draft of the Artificial Intelligence Act in 2021. We believe it is imperative to bring together experts in AI and Law to discuss open questions and regulatory challenges, as well as to develop an interdisciplinary research agenda. The proposed bridge meeting targets two groups of audiences: (i) AI researchers who are interested in building AI systems with enhanced accountability, fairness, privacy, safety, and transparency subject to various regulations, and (ii) legal scholars who are interested in extending or amending current legal and regulatory approaches for emerging AI technologies. We envision that the proposed bridge meeting will facilitate interdisciplinary dialogues, connect AI and Law researchers for potential collaborations, and lay a solid foundation for community building.
2020-21 Catalyst Project Grants
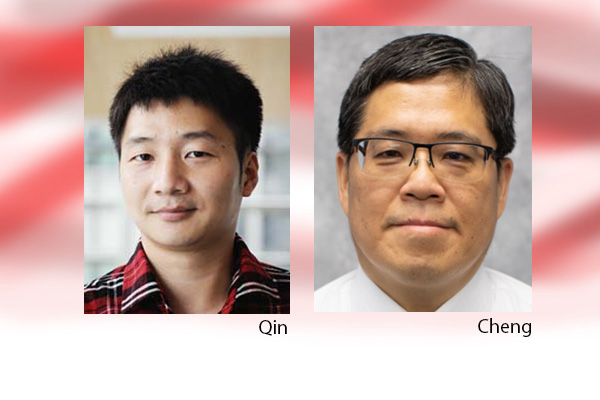
Rongjun Qin (Civil, Environmental, & Geodetic Engineering), Chin-Meng Cheng (Civil & Environmental Engineering)
We can take pictures of a black hole 55-million light-years away, while barely a few meters or less under our feet. The subsurface sources provide more than 80 percent of total U.S. energy needs today, while sensing subsurface for applications such as mining and rare earth element exploration and earth sciences are practically challenging. The current practices rely on expensively drilled boreholes, laborious sample collection at depth, and time-consuming lab analysis with specialized equipment. To allow sample collection, the borehole must be of a certain size (at least 8-10 inches in diameter), and the logistics and cost involved in the complicated sample collection and analysis processes limit the resolution and location of the subsurface data to be collected. If analysis and small-size sensors can be developed to enable onsite and even probes for in-the-borehole analysis, this could enable smaller boreholes with much-reduced cost, and highly selective sample collection with much enhanced efficiency for subsurface exploration. We propose a proof-of-concept study to validate the feasibility of using low-cost hyperspectral cameras, coupled with machine learning and image processing techniques, to identify useful mineral contents and rare earth elements in a borehole environment. The proposed study, if successful, will drive the development of onsite analysis tools and borehole cameras for efficient explorations of the subsurface with much-reduced cost. This project is interdisciplinary, and it requires expertise in sensors for camera design, machine learning for image analysis and environmental and geo-chemical analysis for ground-truthing the results.