TDAI provides funding and other support for interdisciplinary, big data-enabled research teams at Ohio State who leverage AI methods and other data science methodologies to address societal challenges across domains. Awards include up to $50,00 to obtain preliminary data and develop proofs of concept that result in a competitive extramural grant application. In funding awards, special consideration is given to projects that address responsible data science; health and well-being; the environment, climate, and sustainability; and foundations of AI and data science.
Since 2020, TDAI has partnered with other research entities at Ohio State with shared goals to support teams in strategic areas such as AI and health, and climate data science.
If you are wondering how to apply to receive this award, you can find out more information here.
2024-25 Research Pilot Grant Recipients
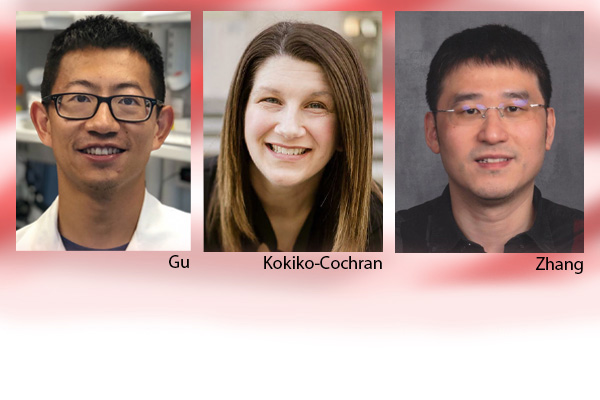
Bin Gu (Neuroscience), Olga Kokiko-Cochran (Department of Neuroscience, Chronic Brain Injury Faculty), Ping Zhang (TDAI Core Faculty, Biomedical Informatics and Computer Science & Engineering)
Traumatic brain injury (TBI) is a major global health issue, with posttraumatic epilepsy (PTE) being one of its most devastating consequences. Despite the identification of EEG biomarkers associated with TBI, there is no consistent, reliable method to predict which individuals will develop PTE. Current EEG analyses are time-consuming, biased, and lack interpretability, underscoring the need for an automated, interpretable deep learning approach. This project aims to develop a deep learning algorithm to predict PTE risk using early post-traumatic EEG data collected from genetically diverse mice. We will apply a transformer-based model to identify hidden features in EEG recordings, enhancing prediction accuracy and interpretability without requiring expert input.
This approach will significantly improve preclinical drug screening for PTE and offer a proof of concept for translating deep learning models to clinical settings, where EEG is routinely used in intensive care units to monitor TBI patients. The study’s innovations include analyzing longitudinal EEG data from a novel animal model, developing the first transformer-based deep learning method for PTE prediction, and using attention weights to interpret the model's behavior. This interdisciplinary effort combines animal models, electrophysiology, and deep learning, bringing together expertise in neuroscience, signal processing, and AI to address an urgent need for predictive tools in TBI and epilepsy research.
Award Amount: $25,000
Chronic Brain Injury Amount: $25,000
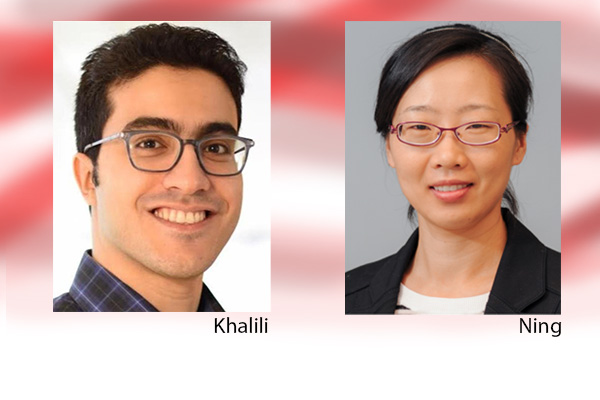
Mahdi Khalili (Computer Science & Engineering), Xia Ning (Biomedical Informatics, College of Medicine, and Computer Science & Engineering, College of Engineering)
While multi-modal machine learning models have shown promise in medical diagnosis, interpreting how different data modalities interact to influence predictions remains a challenge. Existing models typically process each modality separately and then combine the information, making it difficult to understand cross-modal interactions. Transformer-based models, which have revolutionized natural language processing, have been applied to medical diagnosis, but their second-order attention mechanisms are insufficient for fully capturing cross-modal interactions. This project aims to develop an interpretable multi-modal machine learning framework by introducing a "higher-order attention mechanism," which assigns attention scores to groups of tokens across multiple modalities. This will improve both the model's performance and interpretability by enabling a clearer understanding of how modalities interact in the decision-making process.
Furthermore, the proposed framework addresses the trade-off between fairness and accuracy in medical diagnosis, a common issue in machine learning models, particularly in predictions related to demographic biases. By integrating higher-order attention with meta-models, which simplify complex models into more understandable components, our framework will enable clinicians to better interpret predictions, identify key factors influencing disease risk, and recognize sources of prediction disparity. This approach will ultimately enhance the transparency and fairness of machine learning applications in medical contexts, improving both diagnostic accuracy and equity in healthcare decision-making.
Award Amount: $50,000
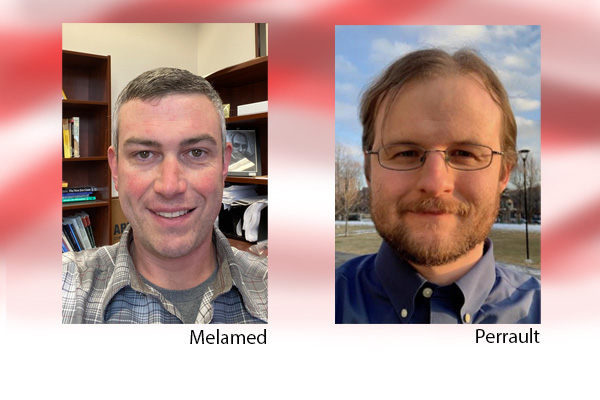
David Melamed (Sociology), Andrew Perrault (Computer Science and Engineering)
Many global challenges, such as climate change and pandemics, are collective action problems where individual interests conflict with group welfare. A potential solution to these dilemmas is the presence of a sanctioning institution that enforces collective participation, with previous research suggesting that sanctions can encourage cooperation. In this proposal, we explore the potential of Artificial Intelligence (AI) as a sanctioning institution. Specifically, we ask whether AI can effectively encourage greater contributions to social dilemmas by applying sanctions based on procedural fairness. AI is perceived as more impartial than humans, and modern systems like large language models can process complex contextual data and make moral judgments similar to human decision-making. We hypothesize that, if AI applies fair and contextually informed procedures, its decisions will be perceived as fair, leading to more sustainable collective action.
Additionally, we aim to collect survey data on collective action and use AI to learn how to optimally allocate participants to groups to maximize collective outcomes. We will compare AI's group allocations to several baseline methods, including random allocation and human-selected groups. This project will shed light on how AI can be leveraged not only to enforce cooperation but also to enhance the effectiveness and sustainability of collective action, offering insights into its potential to address global challenges.
Award Amount: $47,001
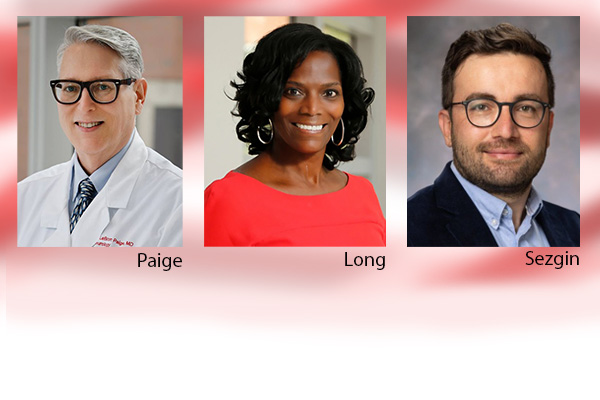
LeBron Paige (Neurology), Emre Sezgin (College of Medicine), Lucretia Long (Neurology)
Epilepsy affects about 1.2% of Americans and is associated with unpredictable seizures that pose significant risks. Traditional seizure management options, such as watchful waiting or calling 9-1-1, have been supplemented by a range of new rescue therapies, including medications and implantable devices. However, these options have added complexity to pre-hospital seizure management, requiring effective initial and ongoing assessment, which can be overwhelming for patients and caregivers. To address this, the Acute Seizure Action Plan (ASAP) has been proposed, but studies have shown that paper-based ASAPs do not effectively reduce emergency room visits or inpatient utilization. We propose a more dynamic solution: an AI-based decision-support chatbot, EpiBot, which would interact with patients and caregivers in real time during a seizure to guide appropriate rescue treatment decisions, improving the timeliness and accuracy of care while reducing unnecessary high-cost interventions.
The proof-of-concept project aims to develop a prototype of EpiBot and use it to inform the development of a next-generation version, guided by feedback from patients and caregivers. By demonstrating the potential clinical impact and cost savings, the project will position EpiBot for future funding and development, including possible support from the Patient Care Innovation Award, NIH/NSF, and other epilepsy-related organizations. In the long term, EpiBot could expand beyond decision support to serve as a platform for additional epilepsy-related functionalities, such as seizure diaries, medication reminders, and educational tools. With integration into epilepsy-focused smart communities, EpiBot could increase its visibility and acceptance, potentially transforming acute seizure care and enhancing the overall management of epilepsy.
Award Amount: $50,000
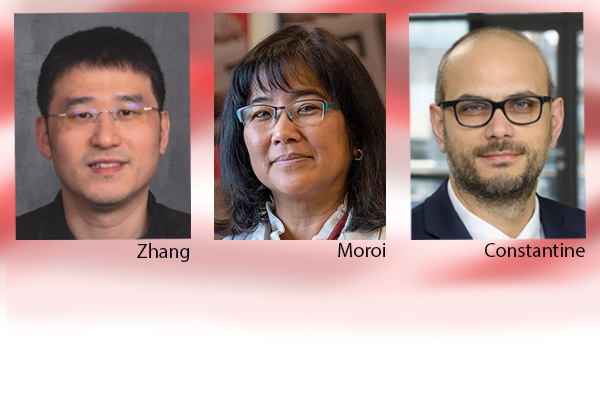
Ping Zhang (TDAI Core Faculty, Biomedical Informatics and Computer Science & Engineering), Sayoko Moroi (Department of Ophthalmology and Visual Sciences), Maged Costantine (Obstetrics and Gynecology)
Preeclampsia (PE) is a serious pregnancy disorder characterized by hypertension and proteinuria after 20 weeks of gestation, affecting about 8% of pregnant women. It can lead to organ damage, maternal mortality, and risks to the baby. Early detection of women at risk for PE offers the opportunity for preventive therapies, such as low-dose aspirin, which has been shown to reduce the incidence of preterm PE. However, predicting PE remains challenging due to the low positive predictive value of current models, which largely rely on Electronic Health Records (EHRs). Furthermore, while retinal microvascular changes have been observed in women with PE, these images are often not included in predictive studies, limiting the understanding of the disease's progression and its vascular manifestations.
This proposal aims to develop deep-learning-based models that incorporate both EHR data and retinal fundus images to improve early-onset PE prediction. By using multi-modal data from 16 weeks of gestation onwards, the model will generate a PE probability for the subsequent 2 weeks, dynamically progressing until delivery. When high-risk women are identified, the system will trigger alerts and recommend early interventions, such as low-dose aspirin, to clinicians. This integrated, multi-modal approach promises to enhance early detection and allow for more targeted, effective management of PE during pregnancy.
Award Amount: $50,000
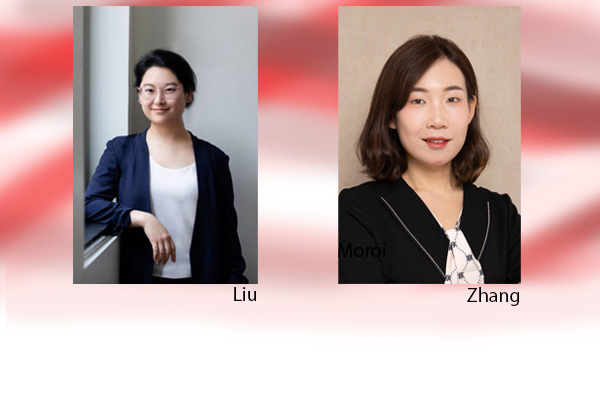
Xueru Zhang (Computer Science and Engineering), Bingjie Liu (School of Communication)
Machine learning (ML) and artificial intelligence (AI) are increasingly used in automated hiring to improve efficiency and achieve diversity, equality, and inclusion (DEI) goals. However, existing studies show that AI tools can unintentionally reinforce biases, as seen in Amazon’s automated resume screening system and AI technologies assessing candidates’ video interviews, which have shown gender and racial biases. Despite efforts to address these issues through fairness notions and de-biasing techniques, challenges remain. First, there is no clear guidance on which fairness notion best applies to hiring, and conflicting fairness criteria can harm both employers and employees. Second, current de-biasing approaches focus on the algorithmic side but neglect the human-AI interaction, leaving the alignment of AI hiring decisions with recruiter and employee expectations unclear. Finally, most solutions address only one stage of the hiring process, whereas fairness should span the entire recruitment pipeline.
This project aims to address these challenges by developing user-aligned, fair automated hiring tools. The team will train ML algorithms using existing fairness techniques, then conduct human subject studies to assess the perceptions of recruiters and applicants towards these de-biased tools. These findings will help determine the most suitable fairness notions for automated hiring and inform the design of algorithms that ensure fairness throughout the hiring process, from resume screening to interviews. This project’s results will not only promote fairer AI recruitment tools but also provide valuable insights to both the ML and HR communities, guiding the development of more equitable and effective hiring practices. The project leverages the interdisciplinary expertise of the team, with PI Liu specializing in human-computer interaction and PI Zhang focusing on algorithm design, ensuring a comprehensive approach to both technical and human-centered aspects of fairness.
Award Amount: $50,000
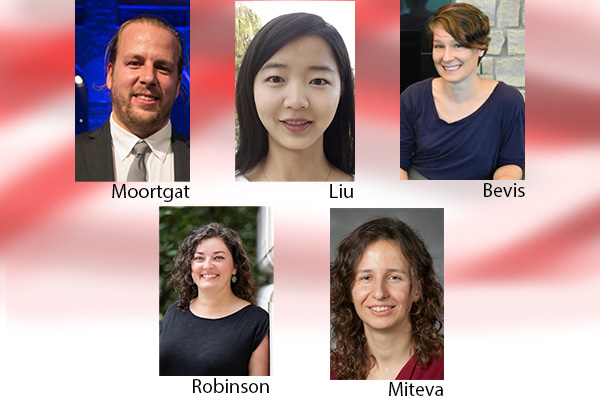
Joachim Moortgat (School of Earth Sciences), Yanlan Liu (School of Earth Sciences), Daniela Miteva (Sustainable Development and Economy at the Department of Agricultural, Environmental, and Development Economic), Leah Bevis (Department of Agricultural, Environmental and Development Economics), Amanda Robinson (Political Science)
Tropical forests store a significant portion of global carbon and are vital in mitigating climate change. However, deforestation—largely driven by rural populations clearing land for agriculture—remains a major source of greenhouse gas emissions. Balancing agricultural development with forest protection is crucial, yet many tropical regions suffer from data scarcity, particularly in Africa, Latin America, and Southeast Asia, where remote communities often lack sufficient on-the-ground data. To address this, we propose using high-resolution imagery from the European Sentinel-2 satellites, combined with AI-driven remote sensing and survey data, to enhance understanding of deforestation dynamics and inform policy.
The research will first assess the impact of increasing women’s participation in local-level natural resource management committees in Malawi, using remote sensing to measure forest outcomes. Additionally, we will explore how agricultural intensification and expansion interact with deforestation across sub-Saharan Africa, aiming to identify systems where intensification reduces deforestation. The findings will provide actionable insights on promoting rural welfare while safeguarding forests, using both fieldwork and big data analytics to support climate solutions in data-scarce regions.
Award Amount: $35,000
Sustainability Institute Amount: $15,000
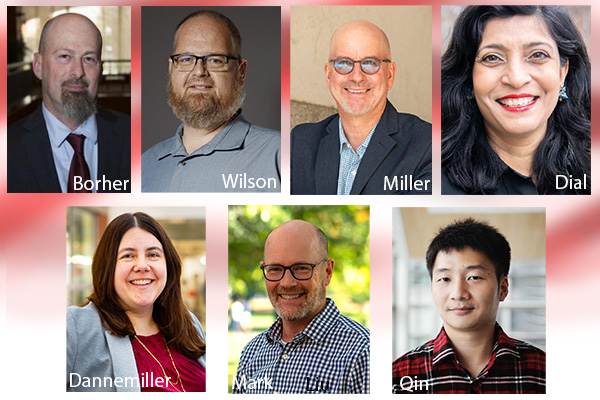
Rongjun Qin (Civil, Environmental, & Geodetic Engineering and Electrical & Computer Engineering), Gil Bohrer (Civil, Environmental, & Geodetic Engineering), Harvey Miller (Geography), Bryan Mark (Geography), Aaron Wilson (Atmospheric Science), Karen Dannemiller (Environmental Health Science), Aparna Dial (Facilities Management and Sustainability)
Grand challenges in modern research require multi-disciplinary collaborations supported by cohesive, federated data infrastructures. According to NSF’s "Big 10 Ideas," these infrastructures should engage research communities at a macro level to enable fundamental discoveries. However, institutions like The Ohio State University (OSU) often face challenges in leveraging the wealth of data generated across disciplines, as researchers are unaware of datasets being created just a few hundred feet away. This limits opportunities for interdisciplinary collaboration and data-driven innovation.
To address this, we propose the OSU-DT-Engine, a collaborative platform designed to aggregate and share digital twin (DT) data across OSU. This platform will centralize the streaming and sharing of multi-scale, geospatially coherent, and near-real-time data, enabling seamless data access and knowledge exchange. By creating a centralized system for searching, indexing, and contributing high-quality geospatial content, the OSU-DT-Engine will foster collaborations and drive transformative innovations across research teams at OSU and beyond.
Award Amount: $25,000
Sustainability Institute Amount: $25,000
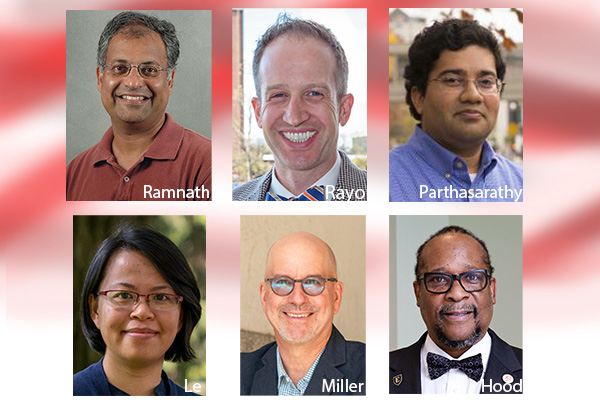
Mike Rayo (Cognitive Systems Engineering), Harvey Miller (Geography), Srini Parthasarathy (Computer Science & Engineering and Biomedical Informatics), Rajiv Ramnath (Computer Science & Engineering and College of Engineering)
Fostering inclusive and meaningful community engagement in urban planning is a persistent challenge, particularly as the world becomes more urbanized and interconnected. Achieving sustainable, socially just, and environmentally friendly communities requires more polycentric decision-making, transparency, and flexibility, especially in the face of crises like extreme weather and pandemics. Effective community co-planning can help bridge knowledge gaps and give stakeholders greater agency, but it is often hindered by resource constraints and engagement barriers. Our hypothesis is that improving dialogue between planners and communities will increase participation in governance, such as attendance at public meetings and local organizing roles.
To address these challenges, we propose the Public Engagement to Re-imagine Community Co-planning (PERCC) platform—an integrated set of digital and analog tools aimed at enhancing community-engaged co-planning for urban sustainability. By utilizing AI and machine learning, PERCC will facilitate knowledge coproduction, translating community evidence and stories into accessible formats and returning them to the community. The platform will overcome barriers of time, space, and technology by integrating both digital and low-tech communication methods, helping to engage a wider range of participants. We will test the PERCC toolkit in a co-planning process in the Near East Side of Columbus, Ohio, with the goal of scaling its lessons to other communities nationwide.
Award Amount: $49,882
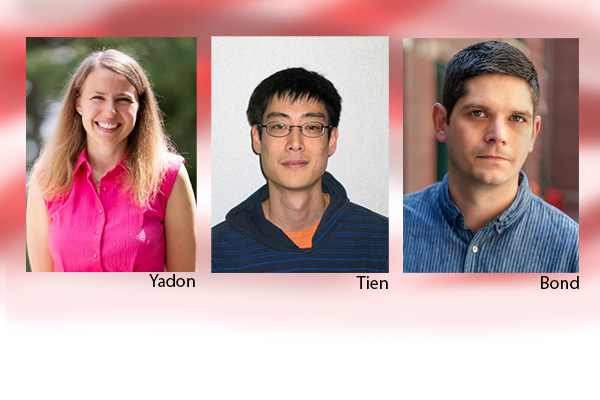
Joe Tien (Department of Mathematics), Nicole Yadon (Political Science), Robert Bond (Political Science and School of Communication)
Online platforms play important roles in shaping the discourse on race in America. In this project, we will develop a structured ‘sentinel’ approach that leverages the community structure of social media networks together with natural language processing techniques to monitor online discourse about race on multiple social media platforms. Given increasing rates and high-profile incidents of attacks against various groups in recent years – including Jewish, Asian, and Black Americans – we are primarily interested in examining how racist content spreads via online platforms. The project will produce outcomes of interest to both academics and lay audiences. This includes a structured dataset allowing contextualization of content relating to race, with a particular focus on anti-Semitic, anti-Asian, and antiBlack content. Analyses of these data will include (i) identifying what types of racist content find widespread engagement (as opposed to being confined to e.g. extremist communities), (ii) studying cross-platform pathways for dissemination of racist content, (iii) documenting the influence campaigns to drive wedges between different demographic subgroups, and (iv) identifying the spread of racist content earlier in information flows with an eye towards mitigating its effect and spread.
Award Amount: $38,901
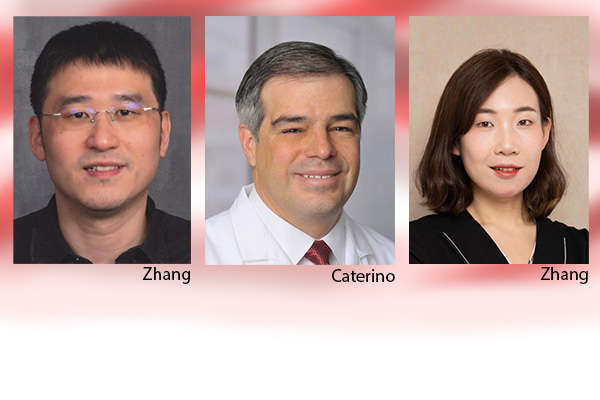
Xueru Zhang (Computer Science and Engineering), Ping Zhang (TDAI Core Faculty, Biomedical Informatics and Computer Science & Engineering), Jeffrey Caterino (Emergency and Internal Medicine)
Machine learning (ML) systems have been increasingly developed and used for recognizing diagnoses based on their clinical manifestations. Concerns have arisen that these systems may exhibit discrimination and be biased against certain patient groups. For example, it has been shown that ML for predicting atherosclerotic cardiovascular disease (ASCVD) risk may have racial biases1-3, skin-cancer detection models may fail to detect early-stage disease in patients with dark skin4; medical resources may be disproportionately allocated among patients with different socioeconomic status1, etc. It is thus critical to ensure ML models developed and used in clinical applications are fair. Although various methods have been proposed for learning fair predictors, they have largely relied on the assumption that the training and testing data (i) come from the same feature and label spaces so that the models only focus on a closed set of previously seen diseases; (ii) have static and identical data distribution over time. Unfortunately, this assumption is commonly violated in practice where disease prevalences are constantly changing and never-before-seen diseases (e.g., COVID-19) may appear suddenly. As a result, ML models that are fair and accurate during training may violate fairness, fail to identify new diseases, and even make wrong predictions during deployment.
Award Amount: $50,000
2022-23 Award Recipients
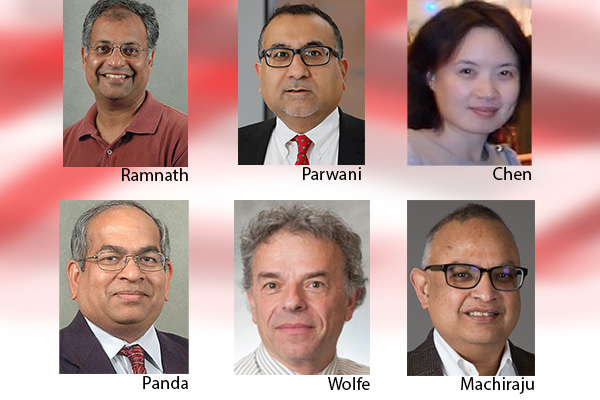
Jian Chen (Computer Science & Engineering), Anil Parwani (Pathology and Biomedical Informants), Raghu Machiraju (Biomedical Informatics), Dhabaleswar K. Panda (Computer Science & Engineering), Rajiv Ramnath (Computer Science & Engineering), Jeremy Wolfe (Harvard, Asset Protection)
This project aims to improve diagnostic accuracy in pathology by developing a human-AI teaming model to assist pathologists in interpreting whole slide images (WSIs), which are large, high-resolution images used for disease diagnosis. Pathologists are facing increasing workloads, and current AI solutions often function as "black-box" systems that lack interpretability, making it difficult for pathologists to understand or trust AI decisions. This proposal envisions a system where AI serves as a cognitive aid, complementing the pathologist's expertise by providing personalized, behavior-aware assistance.
The immediate goal is to collect pilot data to assess the feasibility of this approach, with two main aims: (1) to analyze pathologists' reading behaviors by recording data on their interactions with WSIs, including mouse, eye, and head-tracking, to identify differences across levels of expertise, and (2) to explore novel, unobtrusive AI interventions—such as blink-based visual guidance—to direct the pathologist's attention for faster and more accurate diagnoses. The long-term goal is to create a system that leverages AI as a safety-net, offering personalized, context-aware support to pathologists based on their cognitive profiles and workflow, ultimately improving diagnostic decision-making and enhancing human-AI collaboration in medicine.
Award Amount: $50,000
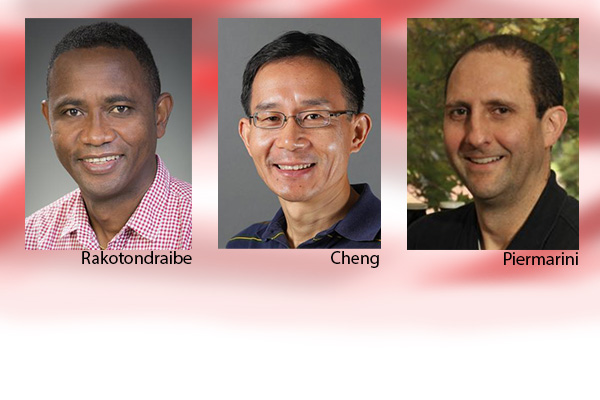
Xiaolin Cheng (Pharmacy), Peter Piermarini (Environmental Sciences), Liva Rakotondraibe (Pharmacy)
Award Amount: $10,000
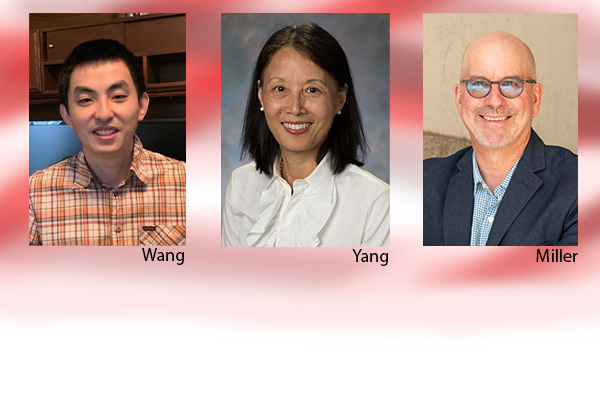
Jingzhen Yang (Epidemiology), Harvey Miller (Department of Geography), Yang Wang (Computer Science & Engineering)
This pilot study aims to reduce traffic-related injuries and fatalities among teen drivers by exploring the impact of personal factors and built environmental features on risky driving behaviors. Teen drivers are disproportionately involved in crashes, with a crash rate much higher than older drivers, leading to significant medical and productivity costs. Previous research has primarily focused on personal behaviors like driving errors and risk-taking, but little is known about how built environments—such as street designs and road features—contribute to teen driving risks.
The study has two main goals: (1) to assess how built environmental features (e.g., street images and road networks) relate to risky driving behaviors like speeding and hard braking, and (2) to identify and predict high-risk driving hotspots for teens. By identifying the environmental factors that increase risk, the study will provide essential data for future research and interventions aimed at reducing teen driving crashes. This research also aims to explore the role of greenery and other environmental factors in improving road safety for teen drivers.
Award Amount: $50,000
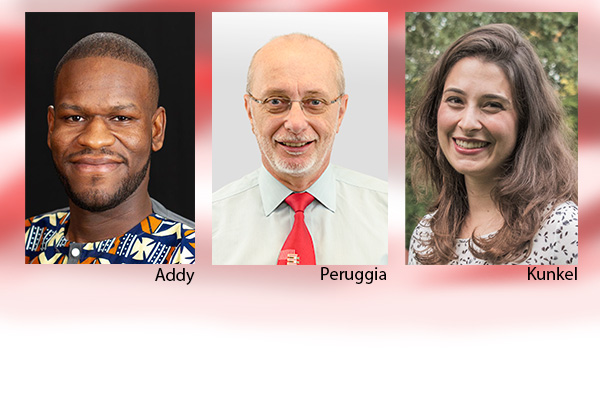
Mario Peruggia (Department of Statistics), Shadrick Addy (Department of Design), Deborah Kunkel (Statistics)
Virtual reality (VR) technology enables users to immerse themselves in realistic scenarios that emulate real-life situations, using head-mounted displays and hand-held controllers. We will consider VR experiences that have beneficial implications for society. Co-PI Addy has collaborated in the design of a vast array of such experiences, ranging from VR training of social workers and medical residents to educational VR immersion in the soil ecosystem from the perspective of an underground creature, to VR embodiment and preservation of tangible and intangible cultural artifacts in war-torn areas of the world. We will collect real-time behavioral measurements (user position, gaze direction, etc.) from participants through the VR headset and controllers. Leveraging the design and data analysis interdisciplinary expertise of our team, we will establish a data-driven approach to inform VR design (and accessibility if hearing, vision, and mobility impediments are present) based on user decisions, supplementing the current use of user survey responses. We are well poised to foster the design of culturally inclusive and realistic VR experiences because of the ethnic and gender diversity of our team that merges the cultural heritages from our birthplaces in Africa, America, Asia, and Europe.
Award Amount: $50,000
2021-22 Award Recipients
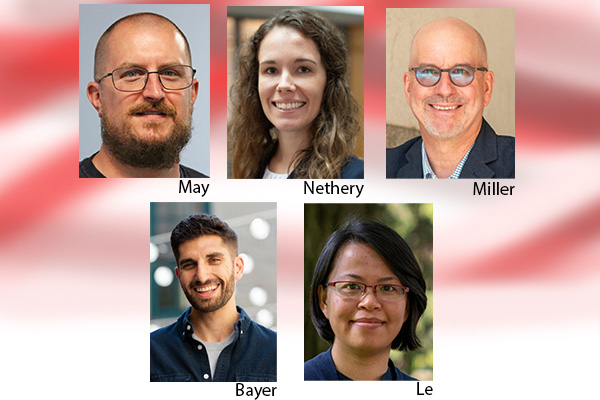
Huyen T. K. Le (Department of Geography), Andrew May (Civil, Environmental & Geodetic Engineering) Joseph B. Bayer (TDAI Core Faculty, Communication), Harvey Miller (Department of Geography), Rachel Nethery (Biostatistics)
Traffic-related pollutants, particularly particulate matter smaller than 2.5 microns (PM2.5), contribute significantly to respiratory and cardiovascular diseases, mental illness, and premature deaths. In 2011, PM2.5 from transportation caused nearly 29,960 premature deaths in the U.S., representing 28% of all anthropogenic PM2.5-related deaths. Although road users spend only 4% of their time in traffic, they experience 8% of daily PM2.5 exposure, with vulnerable populations—such as non-auto users and low-income or minority communities—disproportionately affected. Most studies focus on home-based exposure, neglecting the impact of non-home, traffic-related exposure. To address this, we propose a study to quantify PM2.5 exposure during daily travel, considering spatio-temporal patterns, travel modes (e.g., driving, walking, biking), and socioeconomic disparities in Columbus, OH. The project will integrate environmental, geographical, behavioral, health, and data sciences to estimate short- and long-term health impacts (e.g., respiratory issues, cardiovascular diseases, stress, anxiety).
Research objectives include: (1) measuring on-/near-road PM2.5 using low-cost sensors; (2) generating high-resolution spatio-temporal predictions of PM2.5 concentrations; and (3) quantifying exposure by linking GPS data with PM2.5 levels to assess health outcomes. The results will provide data and models to answer whether on-/near-road air is healthy, guiding evidence-based decisions in transportation planning and land use. Ultimately, this research aims to inform local and regional policies to reduce traffic-related pollution and promote public health and equity.
Award Amount: $46,000
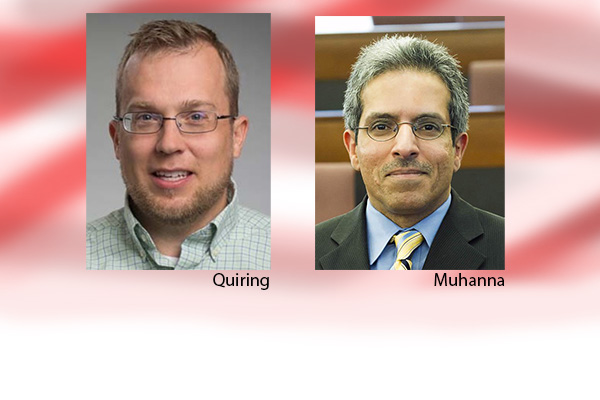
Steven Quiring (TDAI Core Faculty, Geography), Waleed Muhanna (College of Business)
This research project aims to develop advanced catastrophe loss models for hurricanes, focusing on improving risk management for the insurance industry, particularly in the face of increasing frequency and severity of weather-related events. The models will incorporate historical data on weather hazards and building characteristics to predict the number and severity of claims from catastrophic weather events. By using machine learning techniques, the goal is to generate accurate, timely forecasts that can help insurance companies manage their financial exposure and optimize their disaster response strategies. The project partners with Nationwide Insurance, which will provide claims and policy data from recent hurricanes to help validate these models. Additionally, the models have potential commercial applications for both the insurance industry and government emergency management agencies, with interest from companies like Owens Corning, which has a stake in storm-related damage to its products. The research combines expertise in atmospheric science and predictive analytics, particularly in addressing challenges like class imbalance in rare event prediction, to create more effective tools for storm preparation, response, and risk assessment.
The collaborative effort between Professors Quiring and Muhanna builds on previous research in predicting the impacts of hurricanes on infrastructure, such as power systems. The team plans to employ a two-stage random forest modeling approach, shown in past studies to be the most accurate for such predictions while addressing the "class imbalance problem" common in predictive modeling for rare events. This innovative project has the potential to advance decision-making tools that can improve both the operational efficiency of insurance firms and the overall resilience of communities to catastrophic weather events.
Award Amount: $35,000
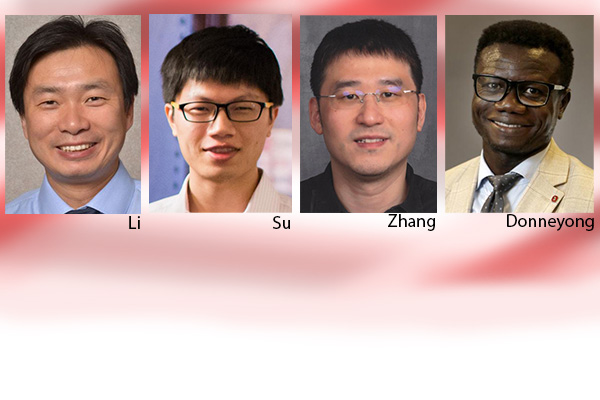
Yu Su (Computer Science & Engineering), Ping Zhang (TDAI Core Faculty, Biomedical Informatics and Computer Science & Engineering), Lang Li (Biomedical Informatics), Macarius Donneyong (TDAI Core Faculty, Pharmacy and Public Health)
This project aims to enhance pharmacovigilance by integrating social media data into the detection of adverse drug events (ADEs). ADEs are a major public health issue, causing a significant number of hospital visits and deaths each year, but current detection methods, such as clinical trials and spontaneous reporting systems (SRSs), have limitations. Clinical trials often miss rare ADEs, and SRSs suffer from under-reporting. Social media data has shown promise in addressing these gaps by detecting rare events, and quality-of-life issues like insomnia, and drug misuse, often overlooked by traditional systems.
The project focuses on three key areas: 1) compiling and enhancing social media ADE datasets, 2) developing advanced machine learning and natural language processing (NLP) techniques to improve automated ADE detection, and 3) creating a social media pharmacovigilance monitoring system with streamlined data pipelines from multiple platforms. Interdisciplinary collaboration between AI, biomedical informatics, and pharmacy experts is essential for developing high-quality data annotation guidelines and effective signal evaluation methods. The project is part of a broader initiative to address drug safety issues and establish OSU as a leading center for pharmacovigilance.
Award Amount: $51,000
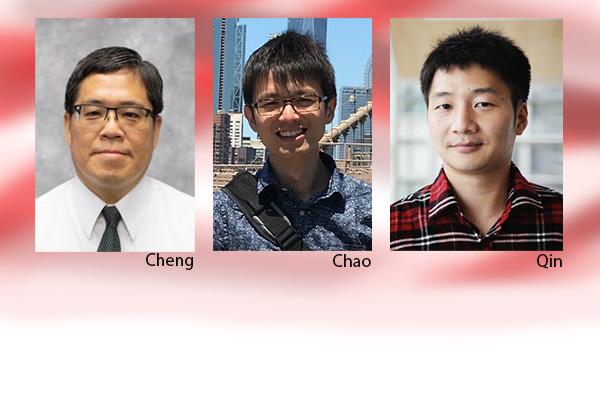
Rongjun Qin (Civil, Environmental, & Geodetic Engineering and Electrical & Computer Engineering), Chin-Min Cheng, Wei-Lun Chao (Computer Science & Engineering)
Award Amount: $10,000
2020-21 Award Recipients
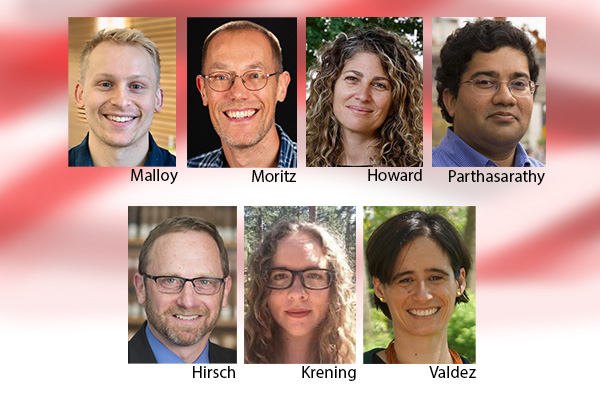
Ines Valdez, Mark Moritz (Department of Anthropology), Dennis Hirsch (TDAI Core Faculty, Moritz College of Law, Program on Data & Governance), Dana Howard (Department of Philosophy), Samantha Krening (Integrated Systems Engineering), Samuel Malloy (Science, Engineering & Public Policy), Srinivasan Parthasarathy (Computer Science & Engineering and Biomedical Informatics)
Through an integrated approach, we will develop a vibrant interdisciplinary community that uses a critical approach to data analytics. This community will design a curriculum in Social, Political and Ethical Dimensions of Data Science, including modules for the data analytics major, a graduate interdisciplinary specialization, and a certificate open to students, faculty, professionals, and broader audiences.
By being led by an interdisciplinary team, addressing head on questions of the broader impact of research, and designing curriculum innovations to prepare students to address social and political dimensions of data science, the project will be highly competitive for extramural funding.
Award Amount: $50,000
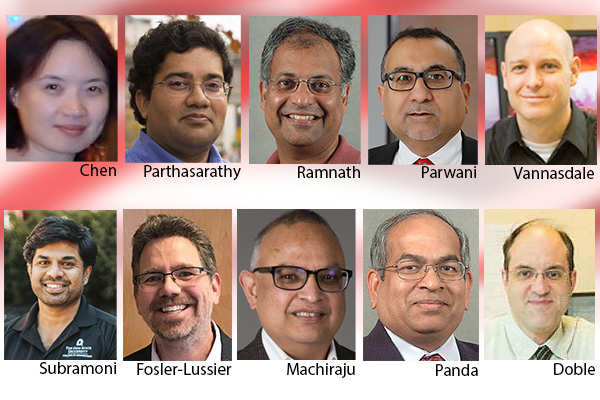
Jian Chen (Computer Science & Engineering), Raghu Machiraju (Biomedical Informatics), Nathan Doble (Optometry, Ophthalmology, and Visual Science), Anil Parwani (Pathology and Biomedical Informatics)
Additional Collaborators: Jose Otero (Neuroscience), Zaibo Li (Molecular Biology and Cancer Genetics Program), David Liebner (Medical Oncology)
Humans and machines collaborate in the analysis of multi-modal, multi-dimensional images in order to extract scientific insights in health and medicine. However, the exponential increase in the complexity, speed and volume of the images has outpaced human cognitive ability. Ground-breaking paradigms in computing and related areas need to be discovered.
A proposal to the NSF-Expeditions program in 2019 did not succeed because gaps were noted in problem definition and the research approaches. Thus, the primary goal of this seed effort is to do the preliminary work needed to write successful proposals to funding agencies, while at the same time making discoveries through proof-of-concepts (POC). Specific outcomes are: (1) POCs and preliminary research data (2) definitions of the research problems (3) team formation, (4) designed pathways for broader impact and participation all leading to (5), a set of compelling proposals to specific funding agencies.
Award Amount: $46,000
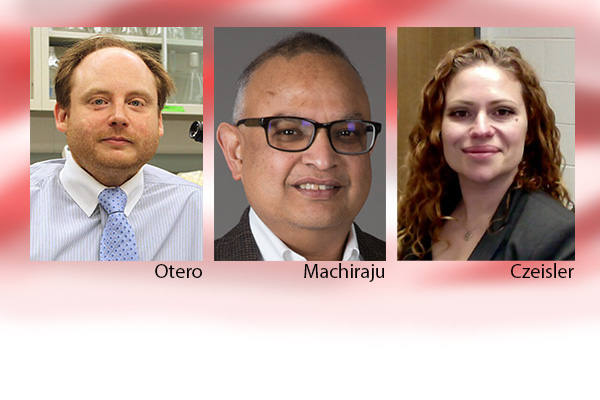
Jose Otero (Neuroscience, Pathology, Neuropathology), Catherine Czeisler (Pathology and Philosophy), Raghu Machiraju (Biomedical Informatics)
The diagnostic workup of brain tumors requires a panel of immunohistochemical stains with a subset of tumors requiring additional molecular testing to reach a diagnostic category recognized by the World Health Organization. In the United States and worldwide, scarce resources are available to perform these tests, so methods that improve pre-test probabilities and decrease false positive results have significant clinical and financial impact. Our long-term goal is to improve and standardize testing and diagnoses for brain tumor patients worldwide by validating new diagnostic workflows using digital imaging, immunohistochemical tests, open-source computing platforms, and machine learning algorithms to improve diagnostic capabilities. The Ohio State University is the first US cancer center to transition to complete whole slide imaging, and therefore by evaluating this pre-existing clinical dataset we are in a unique position to generate a significant, vertical advance in improving diagnostic accuracy in neuropathology with modern Pathology Informatics approaches.
Award Amount: $10,000
Co-funded by: TDAI, Center for Clinical and Translational Science
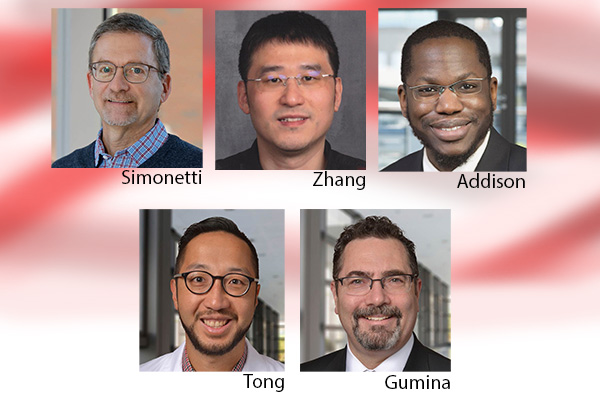
Matthew Tong (Osteopathic Physician and Internal Medicine), Daniel Addison (Cardiovascular Disease Specialist), Ping Zhang (TDAI Core Faculty, Biomedical Informatics and Computer Science & Engineering), Preethi Subramanian (Biomedical Imaging), Orlando Simonetti (Cardiovascular Simonetti), Richard Gumina (Internal Medicine, Interventional Cardiology, Cardiovascular Disease)
The National Quality Strategy in 2011 aims for better and affordable health care through promoting treatment strategies in cardiovascular disease. Large scale registry databases are capable of providing population-level data in an efficient and cost-effective method in improving quality and health care costs. We propose that, through a large cardiac magnetic resonance (CMR) registry database, CMR will provide higher diagnostic accuracy in a cost-effective manner.
Award Amount: $23,200
Co-funded by: TDAI, Center for Clinical and Translational Science, College of Medicine Department of Biomedical Informatics
2017-18 Seed Grant Recipients
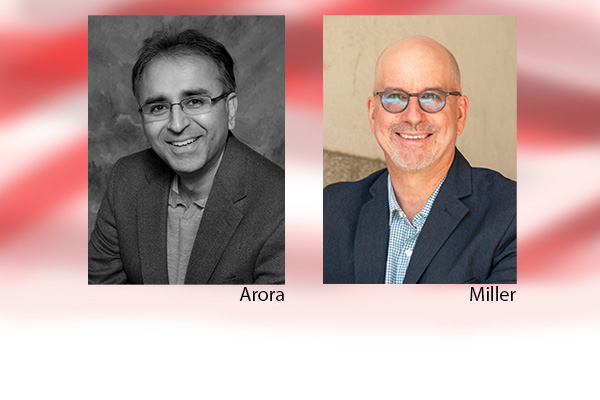
Anish Arora (Computer Science and Engineering), Harvey Miller (Geography)
Persistent, wide-area counting of pedestrians and cyclists is a key yet unserved need in a host of urban applications. Existing data sets in the US are especially limited in their spatio-temporal coverage. We propose to develop and demonstrate low-power, low-cost wireless sensor networks as a basis for addressing this need, and thereby enhance existing data sets as well as support analytics for planning and safety applications. This yearlong effort will pilot a wireless sensor network along the segment of the Olentangy River Road Bike Trail that goes through OSU’s campus and analyze the resulting dataset.
Award Amount: $30,000
Matching support (not required): $32,719
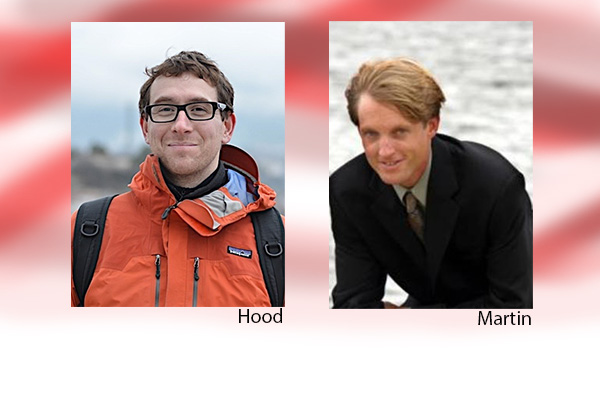
Jim Hood (TDAI Core Faculty, Evolution, Ecology & Organismal Biology), Jay Martin (Agricultural Engineering)
In 2017, Ohio, Michigan, and Ontario launched a plan to reduce phosphorus (P) loading from the Maumee watershed by 40%, requiring significant changes in land use. However, the effectiveness of these efforts remains uncertain due to a limited understanding of how fertilizer application links to riverine P yields. A key gap is the role of in-stream processes in P retention and transformation, which are often oversimplified or ignored in watershed models. These models assume a net-zero effect from riverine P cycles due to unknown parameter values, but evidence shows that up to 60% of available P can be removed in transport, with rivers acting as hotspots for transformations between biologically available and recalcitrant forms. Our research aims to clarify the role of in-stream processes in P cycling within the Maumee River, helping to refine watershed models and improve management strategies. This pilot study will provide data to support future funding and outreach efforts, ultimately enhancing regional management plans. Our objectives are to (1) assess the role of riverine P cycles in P exports and (2) improve watershed models to represent these processes better.
Award Amount: $29,830
Matching Support (not required): $38,265
2016-17 Seed Grant Recipients
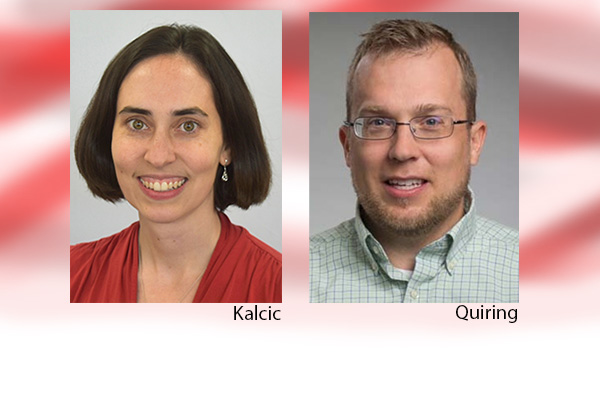
Margaret Kalcic (TDAI Core Faculty, Sustainability Institute), Steven Quiring (TDAI Core Faculty, Geography)
Agricultural lands in watersheds draining to western Lake Erie face increasing pressure to maintain crop yields while reducing environmental impacts, particularly water quality issues linked to nutrient runoff. A harmful algal bloom in 2014 highlighted the dangers of agricultural runoff, which, combined with climatic conditions, led to a water supply crisis in Toledo. In response, U.S. and Canadian stakeholders agreed to a phosphorus load reduction plan to curb algal blooms, but the challenge remains to balance water quality goals with agricultural productivity. Farmers are already adapting to a changing climate with hotter, drier summers and wetter springs, but knowledge gaps persist about how climate extremes like floods and droughts will affect farming. This project aims to support decision-making under uncertainty by identifying optimal conservation practices for agricultural production, considering future climate change scenarios.
Award Amount: $30,000
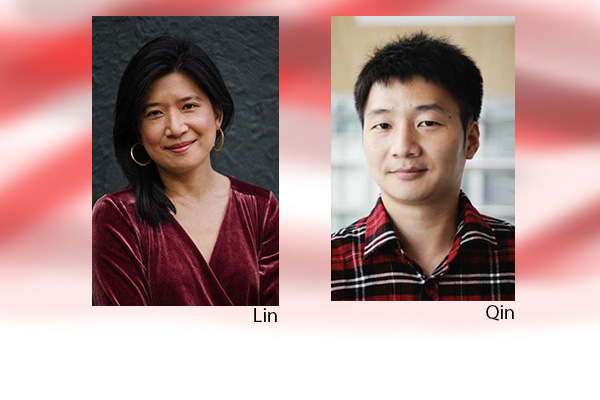
Erin Lin (Political Science), Rongjun Qin (TDAI Core Faculty, Civil, Environmental, & Geodetic Engineering and Electrical & Computer Engineering)
Unexploded ordnance (UXO) from war, such as carpet bombs, cluster munitions, and landmines, pose significant risks to communities, particularly in areas with hidden UXO beneath the ground. Identifying these dangerous areas is a challenge for farmers, clearance teams, and aid organizations. This project aims to develop a remote-sensing method to locate UXO by combining declassified U.S. Air Force data on bomb drop sites with high-resolution terrain and vegetation maps. Machine learning will be used to identify bomb craters, which provide clues about the number of undetonated bombs in each area. By analyzing multispectral imagery, the team will also assess crop activity and yield to estimate the impact of UXO on agricultural land. This method, using data collected since 1998, will provide insights into how UXO has affected arable land over the past two decades, raising awareness of the need for de-mining efforts.
Award Amount: $29,960
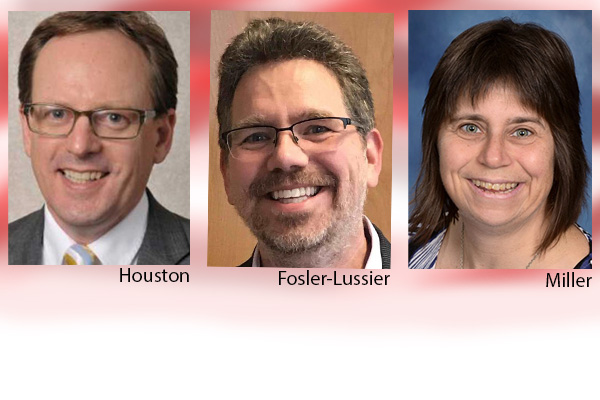
Derek Houston (Otolaryngology, Psychology, and Speech & Hearing Sciences), Amanda Miller (Radiology), Eric Fosler-Lussier (Computer Science & Engineering, College of Engineering, Biomedical Informatics, College of Medicine, Linguistics, College of Arts & Sciences), Andrew Plummer (Computer Science, Linguistics, and Mathematics), Jongmin Jung (Philosophy)
Speech sound production is crucial for communication, and differences in speech development can have significant real-world consequences, as shown in studies from the 1990s. Speech sound disorders affect 2-25% of children, with higher rates among younger children, and a better understanding of their articulatory mechanisms could aid speech-language pathologists in improving communication skills. This project proposes developing new methods to estimate the articulatory configurations and motor control strategies used by infants and toddlers, as well as building models of speech production tailored to young children. Speech-language pathologists from Nationwide Children’s Hospital will provide ongoing input to ensure the models address real-world challenges in treating speech sound disorders.
Award Amount: $26,300.75
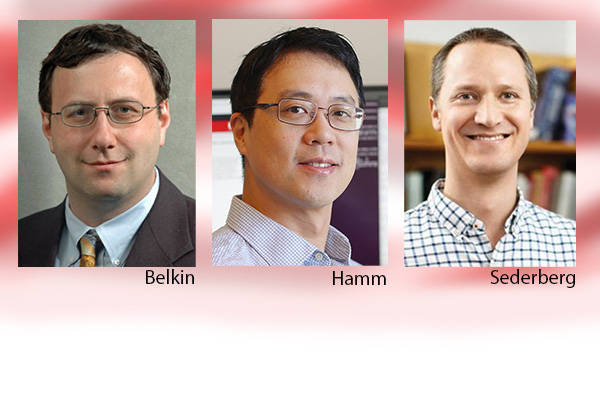
Jihun Hamm (Computer Science & Engineering), Per Sederberg (Psychology), Mikhail Belkin (Computer Science & Engineering)
Understanding how people experience their daily lives and what they learn from real-life events can impact fields like urban planning, public resource management, and personalized health tools. While existing surveys like the American Time Use Survey (ATUS) capture broad patterns, they are limited in scope and duration. Smartphones, however, provide an opportunity to collect continuous, fine-grained data on individuals' behaviors, such as location, motion, and communication, enabling more detailed insights into daily experiences. The researchers aim to use this technology to develop machine-learning algorithms that segment, classify, and predict events from sensory data. A pilot study will be conducted with 20 participants, including both healthy individuals and those with mild cognitive impairment, to identify changes in daily experience that could predict disease and support future memory interventions.
Award Amount: $30,000
2015-16 Seed Grant Recipient
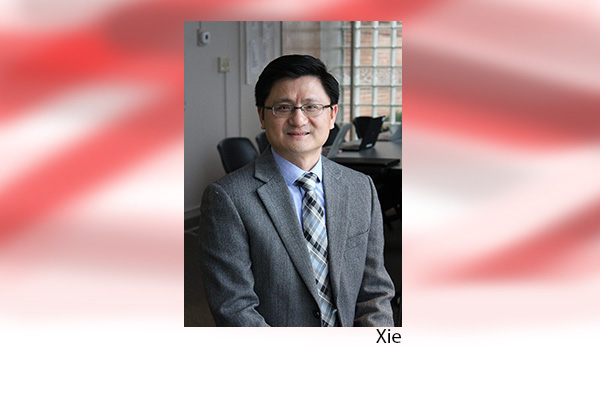
TDAI awarded its inaugural seed grant to Kui Xie, Cyphert Distinguished Professor in the College of Education and Human Ecology’s Department of Educational Studies and the director of the Digital Learning Research Lab.
Award Amount: $24,970