Reflection: Optimism, Skepticism, and Structure: Reflections on the Future Leaders Summit on Responsible Data Science and AI
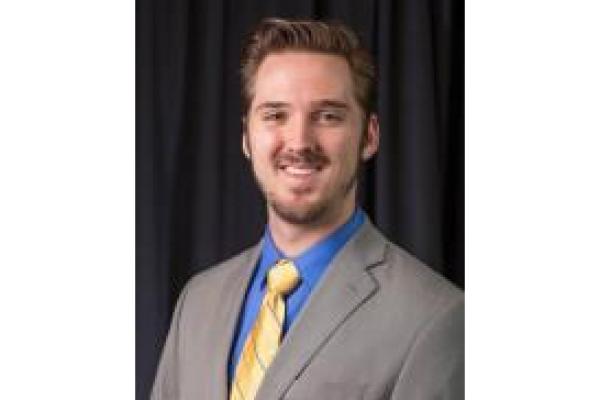
Dane Morey, a GRA in the Cognitive Systems Engineering Lab, has written a reflection on the 2023 Michigan Institute for Data Science (MIDAS) Future Leaders Summit to share with the TDAI community. The reflection summarizes the summit as a whole and what Dane perceives to be the major tendencies in the data science community.
*
Optimism, Skepticism, and Structure
Reflections on the Future Leaders Summit on Responsible Data Science and AI
By Dane Morey
Michigan Institute for Data Science (MIDAS)
April 12-14, 2023
First and foremost, as a relative newcomer to data analytics (coming from a systems engineering background), I was incredibly grateful for the opportunity to connect with, learn from, and speak to so many peers on the leading edge of data science. The Future Leaders Summit hosted by the Michigan Institute for Data Science (MIDAS) comprised a total of 30 doctoral and postdoctoral researchers from 19 different universities around the country. Each attendee gave a brief 10-minute overview of their research and there was a striking variety of domains, methods, and uses of data analytics among the talks. Though our topics were very different, there was a strong communal passion for the responsible utilization of data science and comradery stemming from being in similar stages in our careers. It was relieving to talk with others who, like me, are nearing the end of their academic programs and still grappling with a myriad of options for what comes next. The summit offered ample opportunities for engaging with others in rich conversations about research, careers, and life, and it truly felt like we were laying the foundations for building bridges.
The theme of this year’s MIDAS Future Leaders Summit was responsible data science and AI, which reflected the consensus concern among attendees that data science is often utilized irresponsibly. From examples of algorithms which amplify societal inequities to the lack of understanding surrounding ChatGPT, there was a general uneasiness about how easy it was for data science to negatively impact society and a healthy skepticism that research at large (especially from profit-driven companies) would consistently commit to a responsible approach. Some attendees even perceived a trade-off between “responsible” research practices and doing more work, as if responsible data science was in opposition to the production pressures of organizational expectations.
Despite the clear passion for responsible data science and AI, even if it required “extra” work, there was no clear consensus on what it means for data science to be responsible or how to achieve it. Many attendees had differing and sometimes conflicting ideas on what it means to be responsible with data science. Some emphasized responsibility as a thoughtful development process, while others emphasized responsibility as the outcomes data science has on society. Some spoke of responsibility as making positive impacts or giving back to the community beyond research purposes, while others spoke of responsibility as avoiding unintended consequences or preventing nefarious misuse. Some viewed responsibility as mostly correcting data issues prior to modeling, while others viewed responsibility as mostly evaluating models for societal biases. Though most viewed “black-box” models as insufficient for responsible data science, some advocated for providing explainability or at least transparency for these models, while others advocated for using inherently more interpretable models. Some considered the essence of responsibility to be fairness, yet others considered equity to be the core of responsibility. Although responsibility likely includes many of these elements, the lack of consensus, comprehensiveness, and compatibility among attendees’ notions strongly suggests that responsibility remains an under-specified word within the data science community. There is clearly strong interest, passion, and human-centered motivations to pursue responsible data science, but I remain skeptical that these motivations can be harnessed to make measurable impact without a clearer consensus on what responsibility means and how it can be achieved.
Although the data science community is recognizing that their solutions exist within and impact a broader societal context, an overwhelming majority of attendees seemingly rely only on data science solutions. Dr. Jagadish, Director of MIDAS, strongly emphasized how taking a strictly data-driven approach can impede rather than advance societal equity. All models implicitly assume that the future will be like the past. When the data, features, access, and outcomes of the past are biased, it necessarily requires the intercession of people to be the driving force behind equity and ensure that the future is not like the past. Yet, despite recognizing that algorithms are fundamentally insufficient (or contrary) to equity, Dr. Jagadish remained optimistic that algorithmic decision-making was superior to human decision-making if algorithms were designed with equity in mind. That is, equitable solutions are achievable through data science alone. Ironically, only people can design algorithms with equity in mind, so a belief that algorithms can be designed by people to adequately address equity issues without a belief that people themselves can address equity issues is contradictory. Similarly, most attendee talks appeared to consider only data science solutions when addressing responsibility.
In contrast, two talks explicitly emphasized that because data science exists within and impacts a broader societal context, the broader societal context can (and should) be included as part of the solution itself. Dr. Tanya Berger-Wolf, Director of the Translational Data Analytics Institute at Ohio State, emphasized the critical need for solutions to connect AI and people together in partnership. I spoke on the critical need for solutions to be designed which support (rather than undermine) the adaptability of frontline practitioners. I think it is no coincidence that the two talks which most explicitly invoked a larger systems perspective came from researchers at Ohio State. As I reflect on the Future Leaders Summit, I am grateful for the confluence of expertise that is available at Ohio State and connected through TDAI that appears to produce a relatively unique perspective in the data science community. In emphasizing the importance of this systemic approach, Dr. Berger-Wolf said, “machine learning isn’t the answer to everything,” to which I would add, “and it is never the whole answer.”
Despite the lack of systemic approaches engaging people as part of the solution, I believe there is cause for optimism. Clearly, the data science community is yearning for their work to have a positive impact in the broader societal context with which they exist. Encouragingly, many of the research presentations I observed are moving towards a more structured approach to data science and away from a strictly data-driven approach. This structure was imposed in a variety of ways, including bounding algorithms to human-understandable features and solutions, leveraging domain knowledge, and utilizing more interpretable algorithms. Importantly, these algorithm structures can be utilized to design richer interactions and partnerships with people, a key component of making people part of the solution. Plus, these approaches are also generating better algorithms (compared to less structured approaches). Therefore, I am optimistic that these trends towards more structure can improve algorithms themselves, improve how well algorithms can interact with people, and ultimately improve how well the joint human-machine system can perform together.