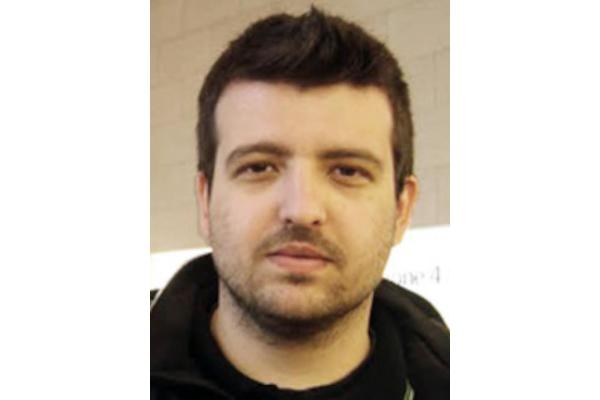
TDAI's Computational Health & Life Sciences community of practice is pleased to present Dr. John Paparrizos (Computer Science & Engineering) on Wednesday, Oct. 25, at 12:00 p.m. in 301* Pomerene Hall. Dr. Paparrizos will give a talk entitled "Next-Generation of Big Multi-Dimensional Data Analytics."
Register for in-person Register for Zoom
*This is an updated location.
Talk Abstract
Today, automated processes, Internet‑of‑Things deployments, and Web and mobile applications generate an overwhelming amount of high‑dimensional data. Meanwhile, computational resources remain limited, and advances in machine learning (ML) create a pressing need to support increasingly expensive and complex analytical tasks. Unfortunately, traditional data management techniques offer limited support for high‑dimensional data, ML tasks, and adaptation to data properties, often resulting in reduced performance. Similarly, due to the difficulty of providing invariances to specific data distortions, applications often resort to inadequate ML methods, reducing their effectiveness.
In my work, I ask how we can address the lack of task‑aware and data‑driven adaptations in data management and ML methods. Specifically, I will discuss two solutions for (i) data representations and (ii) computational methods using techniques to exploit similarities, shapes, densities, and distributions in data. Motivated by the ubiquity of high-dimensional time series, I will first present a method for anomaly detection in streaming data to account for distribution drifts. Then, I will discuss a variance-aware quantization method for indexing high-dimensional data that enables similarity search queries at scale. In both examples, the proposed methods substantially improve performance and accuracy, demonstrating the benefit of designing task-aware and data-driven solutions for large-scale data science applications.