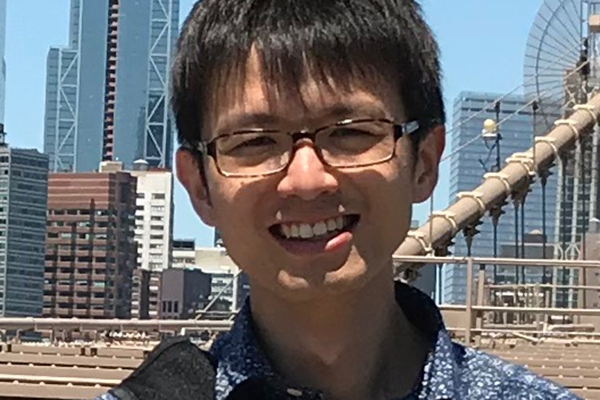
TDAI's Foundations of Data Science & AI community of practice will host a seminar talk by TDAI affiliate Dr. Wei-Lun "Harry" Chao, assistant professor of computer science & engineering, on the topic of federated learning. Further information below.
The event will be on Zoom only.
Abstract:
Modern machine learning models are data-hungry, often requiring a large-scale centralized dataset for training. Collecting such a dataset, however, has significant privacy, security, and ownership concerns. Federated learning is a framework to bypass centralized data, in which individual users keep their data and communicate with a server to train a model collaboratively. While promising, there is still a noticeable performance gap between federated learning and centralized learning, demanding more research efforts to bridge the gap.
In this talk, I will share several interesting, rarely explored, but highly effective aspects to improve federated learning. These include how to aggregate individual users' local models, incorporate normalization layers, and take advantage of pre-training in federated learning.
Federated learning introduces not only challenges but also opportunities. Specifically, the different data distributions among users enable us to learn how to personalize a model. In the second part of the talk, I will share our recent research progress toward personalized federated learning.