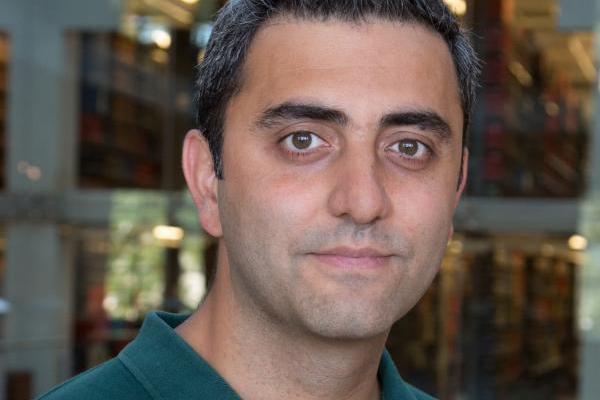
TDAI Core Faculty member Dr. Sam Davanloo Tajbakhsh, Assistant Professor of Integrated Systems Engineering, will give a seminar talk entitled "Stochastic optimization in the absence of unbiased oracles" on behalf of TDAI's Foundations of Data Science & AI community of practice on Monday, February 20, at 3:00 p.m.
The event will be hybrid. Please let us know whether you plan to join us in 301 Pomerene Hall [Register for in-person] or attend remotely via Zoom [Register for Zoom link].
Abstract:
Motivated by multiple emerging applications in machine learning, we consider an optimization problem in a general form where the gradient of the objective is only available through a biased stochastic oracle. We assume the bias magnitude can be controlled by a parameter; however, lower bias requires higher computational cost. For stochastic oracles that do not have bias-variance tradeoff, i.e., oracle's variance does increase when the bias decrease, we propose two algorithms that adaptively change the bias control parameter to exploit the bias-cost tradeoff. We analyze the nonasymptotic performance of the proposed algorithms in the nonconvex regime which will be presented. We also numerically evaluate the performance of the proposed algorithms over two applications on composition optimization and policy optimization for infinite-horizon Markov decision processes.