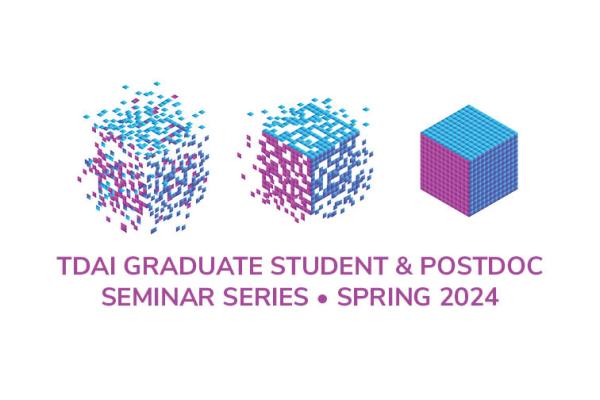
April 10 Speakers
Srishti Gaur
Title: Application of combined data- and knowledge-driven modeling to advance ecohydralogical predictions
Advisor: Darren Drewry
Abstract:
Process-based models are the cornerstone of prediction and knowledge discovery across the environmental sciences. Despite their utility, process-based models are complex tools that require parameterization and re-parameterization as dynamic systems evolve and change over time. Data-driven models, in contrast, have the capability to learn from data but lack the ability to adhere to mechanistic understandings of the underlying physical processes. The next generation of hybrid models is now being developed that integrate process-based and data-driven models in joint architectures, leveraging the knowledge built into process-based formulations with the flexibility and robustness of data-driven approaches.
The process of transpiration from plant leaves and evaporation from the soil surface, known as evapotranspiration (ET), or latent heat flux (LE), is central to quantifying vegetation water use, carbon uptake and the energy balance of the terrestrial surface. The Penman-Monteith (PM) equation is a widely utilized process-based model for estimating ET rates by considering both meteorological drivers and physiological controls.
Here, we present a hybrid model to compute leaf-level latent heat flux which is constrained by the leaf-scale energy budget (i.e. energy conservation). Surface resistance (rs) is the primary unknown element in the PM equation and is a function of multiple factors spanning the meteorological environment of the leaf and the physiological controls the leaf exerts. Semi-empirical models (i.e., Ball-Berry, Leuning) of rs are widely utilized in eco-hydrological and terrestrial biophysical simulation to resolve energy balance and biochemical processes related to the land-atmosphere exchange of carbon, water, and energy. These semi-empirical models for rs are typically based on linear relationships with environmental conditions and require re-parameterization as the phenological and physiological status of the vegetation changes. Machine learning (ML) models offer a potential path to overcome this problem through the development of more flexible, data-driven models.
In this work, we explore the potential of data-driven approaches that leverage our knowledge of the soil-plant-atmosphere continuum to estimate critical land surface variables controlling eco-hydrological processes. This includes purely data-driven approaches to estimate rs, and a set of hybrid approaches integrating biophysical and deep learning formulations. The work presented here utilizes datasets that are global in extent, as well as data collected in focus field efforts to understand the impact of water status and stress on model performance.
Kartik Lovekar
TItle: Estimating differential networks with permutation testing and FDR control
Advisor: Subhadeep Paul
Abstract:
A gene is considered Differentially Expressed (DE) if there is a statistically significant difference in its expression levels between the two conditions. Differentially expressed genes help biologists gain insights regarding mechanisms that govern transcription in phage-host interaction systems. While differential expression of genes (DEG) is a common and useful technique used to find such genes, we propose a method to estimate the differential expression of gene pairs (DEPG), which can help explain how the co-expression of pairs of genes differ between two conditions. We do so by proposing a formal hypothesis test based on a permutation testing framework and compare its performance to an asymptotic test. Our simulations indicate the permutation test yields comparable power to the asymptotic test while controlling the false discovery rate, and has marginally better performance in small samples.
*
TDAI's Foundations of Data Science & AI CoP directors is hosting a graduate student and postdoc seminar series this spring semester over several weeks on Wednesdays, 2:30-4:00 p.m., in 301 Pomerene Hall. Each week, TDAI will host 3-4 speakers who will present for 20-25 minutes with additional time for questions and feedback. The organizers hope this will be a great opportunity for the whole TDAI community to learn about each other's work, help one another refine their presentation skills, and find potential collaborators.
Graduate student or postdoctoral fellows: if you are working with a faculty member affiliated with TDAI, TDAI would love to hear from you about your research. If you are interested in speaking at the series, please email the CoP directors below with a title and abstract of your proposed talk. The directors will select a few proposed talks based on the availability of speaker slots, and if yours is selected, they will get back to you with an invitation. As an interdisciplinary group, TDAI is open to a broad range of topics related to data science and AI.
Current open slots: Wednesdays 2:30 PM - 4:00 PM on April 17.
When you submit your title and abstract, please use the subject line "TDAI Graduate/Postdoc Seminar Series" for your email and send to all of the following addresses: Subhadeep Paul (paul.963@osu.edu), Huan Sun (sun.397@osu.edu), Raef Bassily (bassily.1@osu.edu).