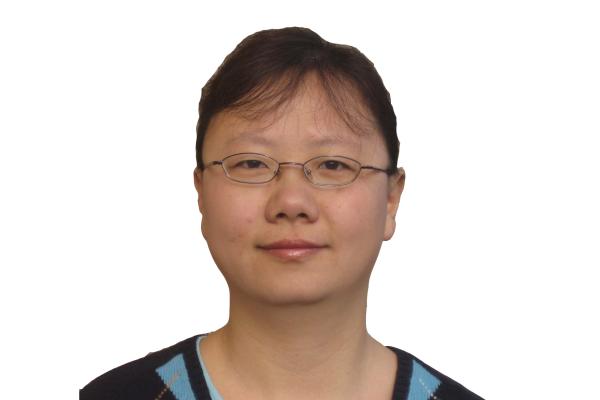
TDAI Core Faculty member Dr. Yingbin Liang, Professor of Electrical and Computer Engineering will present a seminar series talk on behalf of the Foundations of Data Science & AI Community of Practice entitled Reward-free reinforcement learning via sample-efficient representation learning on Thursday, December 8, at 11:00 am on Zoom.
Register for the seminar talk here: https://osu.zoom.us/meeting/register/tJIodOuqqzsqH9zdF6d_I6ZYFBuBQPHBv4oP
Abstract:
As reward-free reinforcement learning (RL) becomes a powerful framework for a variety of multi-objective applications, representation learning arises as an effective technique to deal with the curse of dimensionality in reward-free RL. However, the existing algorithms of representation learning in reward-free RL still suffers seriously from high sample complexity, although they are polynomially efficient. In this talk, I will first present a novel representation learning algorithm that we propose for reward-free RL. We show that such an algorithm provably finds near-optimal policy as well as attaining near-accurate system identification via reward-free exploration, with significantly improved sample complexity compared to the best-known result before. I will then present our characterization of the benefit of representation learning in reward-free multitask (a.k.a. meta) RL as well as the benefit of employing the learned representation from upstream to downstream tasks. I will conclude my talk with remarks of future directions.
The work to be presented was jointly with Yuan Cheng (USTC), Ruiquan Huang (PSU), Dr. Songtao Feng (OSU), Prof. Jing Yang (PSU), and Prof. Hong Zhang (USTC).